
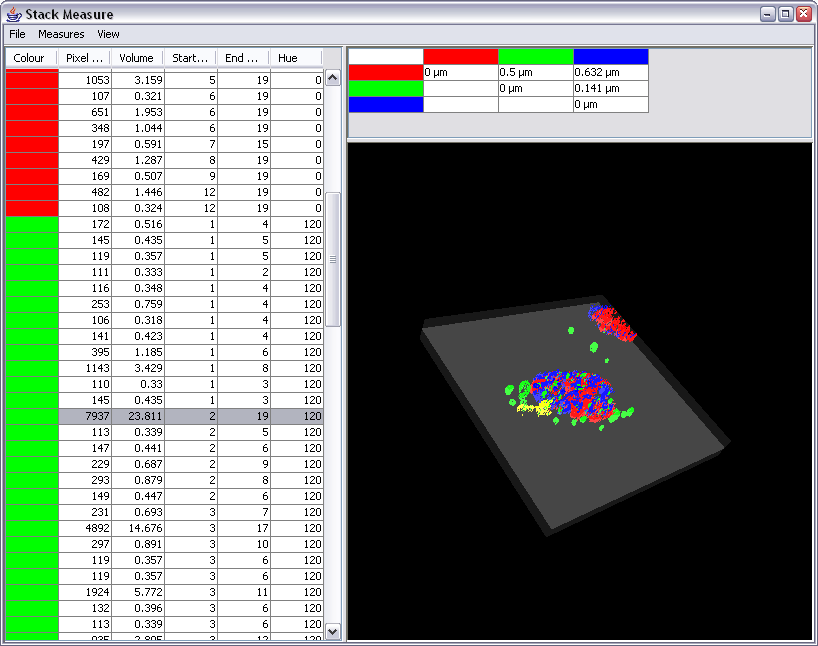
We acknowledge the support of Ministerio de Ciencia, Innovación y Universidades, Agencia Estatal de Investigación, under grant nos TEC2016-78052-R and PID2019-109820RB-I00, MINECO/FEDER, UE, co-financed by European Regional Development Fund (ERDF), ‘A way of making Europe’ (E.G.M., C.G.L.H., A.M.B.), and a 2017 Leonardo Grant for Researchers and Cultural Creators, BBVA Foundation (A.M.B.). We would like specially to mention all the contributors and community partners at the BioImage Model Zoo for the time they have spent to get a cross-compatible model format. Bollmann for including deepImageJ in their tutorials. We would also like to thank NEUBIAS for supporting the project, the NEUBIAS symposium and NEUBIAS and P. Möhl (YAPIC) for the fruitful discussions and enriching feedback about the deepImageJ project. González-Obando (tested the beta-versions), C. Arganda-Carreras (tested the beta-versions), D. ImageJ2: ImageJ for the next generation of scientific image data. Fiji: an open-source platform for biological-image analysis. in Bioimage Analysis Components and Workflows (eds Sladoje, N. Gómez-de-Mariscal, E., Franco, D., Muñoz-Barrutia, A. Usiigaci: Instance-aware cell tracking in stain-free phase contrast microscopy enabled by machine learning. Deep-learning-based segmentation of small extracellular vesicles in transmission electron microscopy images. Nucleus segmentation across imaging experiments: the 2018 data science bowl. Deep-STORM: super-resolution single-molecule microscopy by deep learning. U-Net: deep learning for cell counting, detection, and morphometry. Virtual histological staining of unlabelled tissue-autofluorescence images via deep learning. In Medical Image Computing and Computer Assisted Intervention – MICCAI 2018 – 21st International Conference, Granada, Spain, September 16–20, 2018, Proceedings, Part II 265–273 (Springer, 2018).
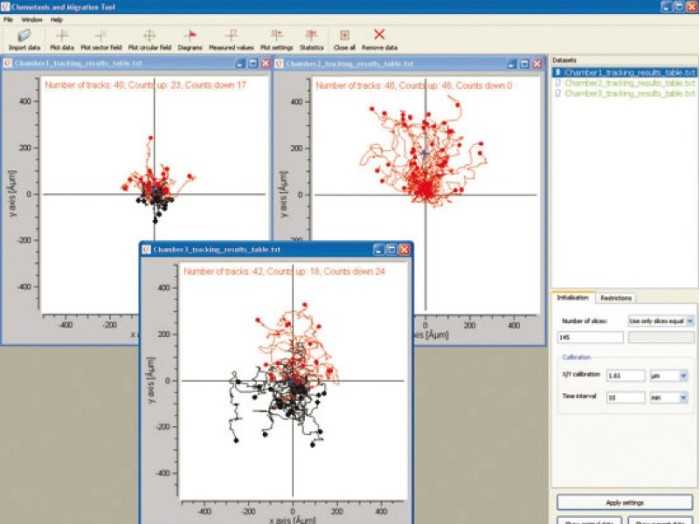
Cell detection with star-convex polygons. NIH Image to ImageJ: 25 years of image analysis. Quantitative digital microscopy with deep learning. Cryo-electron tomography workflows for quantitative analysis of actin networks involved in cell migration. Democratising deep learning for microscopy with ZeroCostDL4Mic. ImJoy: an open-source computational platform for the deep learning era. Ouyang, W., Mueller, F., Hjelmare, M., Lundberg, E.
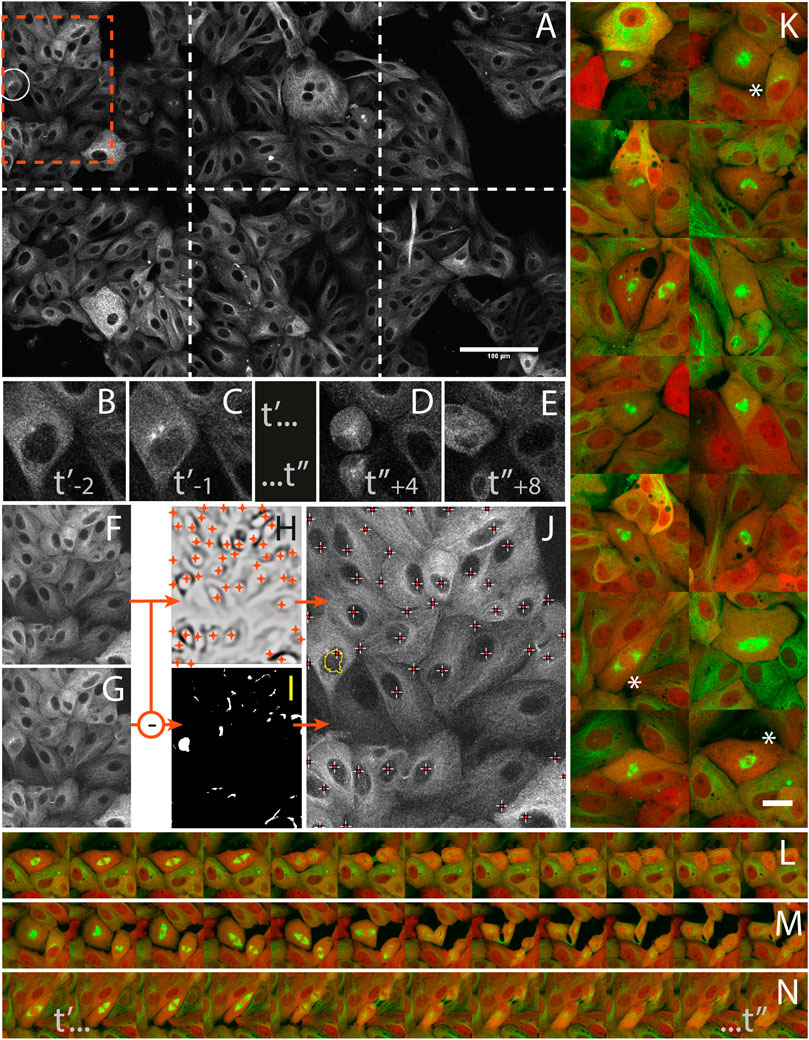
Ilastik: interactive machine learning for (bio)image analysis. DeepClas4Bio: Connecting bioimaging tools with deep learning frameworks for image classification. Inés, A., Domínguez, C., Heras, J., Mata, E. Content-aware image restoration: pushing the limits of fluorescence microscopy. Open-source deep-learning software for bioimage segmentation. The ImageJ ecosystem: Open-source software for image visualization, processing, and analysis. Deep learning for cellular image analysis. A bird’s-eye view of deep learning in bioimage analysis.
